Remote sensing, the art of gathering information about the Earth’s surface without physically being present, has revolutionized our understanding of our planet. At the heart of this technology lie remote sensing sensors, the tireless sentinels that capture the Earth’s electromagnetic signals, revealing its hidden treasures and intricate dynamics.
Types of Remote Sensing
- Based on Platform: This includes satellite-based (like Landsat) and airborne (like UAVs) remote sensing platforms.
- Based on Energy Source: Divided into passive remote sensing, which relies on natural radiation, and active remote sensing, which uses its own signal (like RADAR).
- Based on Imaging Media: Involves optical, infrared, microwave, and other imaging technologies.
- Based on the Regions of the Electromagnetic Spectrum: Utilizes different parts of the spectrum, such as visible, infrared, or microwave, to capture data.
- Based on Number of Bands: Involves multispectral (several bands) and hyperspectral (hundreds of bands) sensors.
Explore these classifications further
This classification is crucial because the platform determines the sensor’s capabilities, such as its spatial, spectral, and temporal resolutions. Here are the main categories based on the platform.
1. Satellite-Based Sensors
- Earth Observation Satellites: These sensors are mounted on satellites orbiting the Earth. They can provide a global perspective and are capable of capturing data over large areas. Examples include the Landsat series, MODIS (Moderate Resolution Imaging Spectroradiometer) on NASA’s Terra and Aqua satellites, and the European Space Agency’s Sentinel series.
- Geostationary Satellites: Positioned in a fixed location relative to the Earth’s surface, these satellites provide constant monitoring over a specific area, making them ideal for weather observation and telecommunications. Examples include the GOES (Geostationary Operational Environmental Satellite) series.
- Polar-Orbiting Satellites: These satellites have a lower orbit and cover the entire Earth by moving from pole to pole. They are ideal for global mapping and environmental monitoring. The NOAA (National Oceanic and Atmospheric Administration) series of satellites is a prime example.
2. Airborne Sensors
- Aircraft-Based Sensors: Mounted on aircraft, these sensors can capture high-resolution data and are often used for detailed mapping, agricultural monitoring, and environmental studies. They offer greater flexibility in terms of scheduling and can be used for targeted missions.
- Drones or UAVs (Unmanned Aerial Vehicles): These are increasingly used for remote sensing due to their ability to fly at lower altitudes, providing ultra-high-resolution imagery. They are particularly useful in precision agriculture, mining, and infrastructure monitoring.
- Balloon-Borne Sensors: While less common, these sensors are used for specific scientific experiments, particularly in atmospheric research. They can provide data from altitudes not typically reached by aircraft.
3. Space Shuttle and ISS-Based Sensors
- Space Shuttle Payloads: Previously, sensors were also mounted on space shuttles for specific missions, such as Earth observation or astronomical studies.
- International Space Station (ISS) Sensors: The ISS hosts several remote sensing instruments used for Earth observation, environmental monitoring, and space-based research.
Each platform offers distinct advantages and limitations. For instance, satellite-based sensors can cover larger areas and are ideal for consistent, long-term monitoring, while airborne sensors offer higher resolution and flexibility but at a higher cost and smaller area coverage. The choice of platform depends on the specific requirements of the remote sensing application, such as the area of interest, required resolution, and budgetary constraints.
Remote sensing sensors can also be broadly classified into two categories: active and passive. Active sensors emit their own energy source, typically in the form of electromagnetic radiation, and measure the reflected or scattered energy to gather information about the target. Passive sensors, on the other hand, detect and measure the energy naturally emitted or reflected from the Earth’s surface.
Active Remote Sensing Sensors
- Radar (Radio Detection and Ranging): Radar sensors emit pulses of radio waves and measure the time delay and strength of the reflected signals to determine the distance, velocity, and scattering properties of the target. Radar is particularly useful for penetrating clouds and darkness, making it indispensable for weather monitoring, land surface mapping, and oceanography.
- LIDAR (Light Detection and Ranging): LIDAR sensors employ laser pulses to measure the distance to the target with high precision. LIDAR is capable of creating detailed 3D maps of the Earth’s surface, with applications ranging from topographic mapping to forest canopy height estimation.
Passive Remote Sensing Sensors
- Radiometers: Radiometers measure the intensity of electromagnetic radiation emitted or reflected from the Earth’s surface across different wavelengths. Radiometric data is crucial for understanding the Earth’s energy balance, land cover classification, and atmospheric properties.
- Spectrometers: Spectrometers measure the spectral signature of the target, providing information about its chemical composition. Spectrometers are widely used in mineral exploration, environmental monitoring, and agricultural applications.
- Imaging Sensors: Imaging sensors capture images of the Earth’s surface, providing a visual representation of the target’s spatial distribution. Imaging sensors operate across various wavelengths, from visible light to thermal infrared, enabling applications such as land use mapping, urban planning, and disaster monitoring.
The choice of imaging media plays a crucial role in the type of data collected and its subsequent applications. Here’s a detailed analysis of the main classifications of remote sensing based on imaging media:
1. Photographic Film:
Technology: Traditional film-based cameras capture images on photographic film.
Characteristics:
- High spatial resolution: Excellent for capturing fine details.
- Limited spectral resolution: Typically restricted to visible and near-infrared wavelengths.
- Analog format: Requires physical development and scanning for digital analysis.
Applications:
- Aerial photography
- Historical mapping
- Geological surveys
- Architectural documentation
2. Digital Sensors:
Technology: Electronic sensors record the electromagnetic radiation directly as digital data.
Characteristics:
- High flexibility: Can capture data across various wavelengths and resolutions.
- Scalability: Can be easily adapted to different platforms and applications.
- Digital format: Enables immediate processing and analysis.
- Wide range of sensor types: CCDs, CMOS, hyperspectral, etc.
Applications:
- Satellite imagery
- Environmental monitoring
- Land cover classification
- Precision agriculture
- Urban planning
- Wildlife conservation
Subdivisions of Digital Sensors:
- Charge-Coupled Devices (CCDs): Highly sensitive sensors with excellent spatial resolution and performance in low-light conditions. Ideal for scientific applications requiring high image quality.
- Complementary Metal-Oxide-Semiconductor (CMOS) Sensors:Lower power consumption compared to CCDs. More suitable for high-frame-rate applications and smaller platforms.
- Hyperspectral Sensors: Capture data across a large number of narrow spectral bands, allowing for detailed analysis of material composition and identification of specific features. Useful for mineral exploration, vegetation mapping, and environmental monitoring.
3. Hybrid Systems:
Technology: Combine film and digital elements for enhanced capabilities.
Characteristics:
- Leverage the advantages of both film and digital sensors.
- Offer high spatial resolution and spectral flexibility in some cases.
Applications:
- Aerial photography
- Topographic mapping
- Land-use change detection
- Cultural heritage preservation
4. Emerging Technologies:
Technology: Continuously evolving advancements in sensor technology offer new capabilities for remote sensing.
Examples:
- 3D Cameras: Capture 3D data of the scene, enabling reconstruction of accurate terrain models and building structures.
- LiDAR (Light Detection and Ranging): Utilizes laser pulses to measure distances and generate high-resolution elevation maps.Ideal for topographic mapping and vegetation studies.
- SAR (Synthetic Aperture Radar): Uses radar pulses to map the Earth’s surface regardless of weather conditions. Useful for disaster monitoring, oil spill detection, and maritime surveillance.
Choosing the Right Imaging Media:
The choice of imaging media depends on the specific needs of the remote sensing application. Factors to consider include:
- Spatial and spectral resolution requirements: Desired level of detail and ability to distinguish different materials.
- Data type: Type of electromagnetic radiation needed for the analysis (e.g., visible, infrared, microwave).
- Platform limitations: Capabilities and constraints of the chosen platform (e.g., satellite, aircraft, drone).
- Budget constraints: Cost of the sensor and associated data processing.
Understanding the different classifications of remote sensing based on imaging media empowers informed selection of the most suitable technology for specific applications. This knowledge allows researchers and professionals to effectively gather valuable information about our planet and its resources, contributing to various fields such as environmental monitoring, land-use planning, and natural disaster management.
Remote sensing sensors utilize various regions of the electromagnetic spectrum (EMR) to capture information about the Earth’s surface and atmosphere. Understanding these wavelengths and their applications is crucial for effective data collection and analysis. Here’s a detailed breakdown of the major EMR regions used in remote sensing:
1. Visible Light (0.4 – 0.7 µm)
- Description: Corresponds to the wavelengths visible to the human eye, encompassing the colours red, green, and blue.
- Characteristics: Sensitive to pigments and surface texture.
- Applications: Photogrammetry, land cover classification, vegetation studies, urban planning.
2. Near-Infrared (0.7 – 1.3 µm)
- Description: Lies just beyond human vision but interacts with chlorophyll, reflecting information about vegetation health and water content.
- Characteristics: Less sensitive to atmospheric scattering compared to visible light.
- Applications: Agriculture (crop health monitoring, irrigation management), forestry, water body delineation.
3. Short-Wave Infrared (1.3 – 3 µm)
- Description: Provides insights into mineral composition and moisture levels.
- Characteristics: Sensitive to hydroxyl groups, useful for mineral identification.
- Applications: Mineral exploration, soil moisture mapping, fire detection.
4. Mid-Wave Infrared (3 – 8 µm)
- Description: Primarily detects thermal emissions, revealing temperature variations on the Earth’s surface.
- Characteristics: Useful for day and night observations, not affected by sunlight.
- Applications: Heat mapping, environmental monitoring, volcanic activity detection.
5. Long-Wave Infrared or Thermal Infrared (8 – 15 µm)
- Description: Focuses on the peak emission of terrestrial objects, offering detailed thermal information.
- Characteristics: Excellent for differentiating materials based on their temperature variances.
- Applications: Nighttime imaging, geothermal activity studies, fire monitoring.
6. Microwave (1 mm – 1 m)
- Description: Utilizes radio waves to penetrate clouds, smoke, and vegetation, enabling all-weather observation.
- Characteristics: Sensitive to surface roughness and dielectric properties.
- Applications: Radar imaging, topographic mapping, soil moisture monitoring, vegetation analysis.
7. Ultraviolet (10 – 400 nm)
- Description: Primarily absorbed by the atmosphere but used for certain Earth observation applications.
- Characteristics: Sensitive to atmospheric composition and certain chemical compounds.
- Applications: Atmospheric studies, ozone monitoring, volcanic plume analysis.
8. X-rays (0.01 – 10 nm)
- Description: High-energy radiation mainly used in astronomy.
- Characteristics: Penetrates dense materials, providing information about their internal structure.
- Applications: Studying celestial objects, black holes, and stellar evolution.
9. Gamma Rays (< 0.01 nm)
- Description: Shortest wavelengths with the highest energy, primarily used in astronomy.
- Characteristics: Highly penetrating, offering insights into extreme cosmic phenomena.
- Applications: Observing gamma-ray bursts, studying the most energetic explosions in the universe.
Understanding the unique capabilities of each EMR region empowers researchers and professionals to:
- Select the most appropriate sensor for their specific remote sensing application.
- Combine data from different regions for more comprehensive analyses.
- Develop new sensors and technologies for more advanced Earth observation.
By harnessing the diverse information contained within the electromagnetic spectrum, remote sensing continues to revolutionize our understanding of our planet and its resources, fostering advancements in various fields like environmental monitoring, disaster management, and resource exploration.
Remote sensing sensors acquire information about the Earth’s surface by recording electromagnetic radiation reflected or emitted from the target. The number of spectral bands they capture significantly impacts the type and detail of information collected, influencing their applications and limitations. Here’s a detailed analysis of this classification:
1. Panchromatic Sensors:
- Description: Capture image data in a single broadband, typically encompassing the visible spectrum (red, green, and blue).
- Characteristics:
- Highest spatial resolution among all sensor types.
- Limited spectral information, unable to differentiate materials based on subtle spectral variations.
- Applications:
- Mapping and terrain analysis.
- General surveillance where detailed color information is not crucial.
- Creating base maps for overlaying other data.
2. Multispectral Sensors:
- Description: Capture data in several discrete spectral bands (usually between 3 and 10).
- Characteristics:
- Moderate spatial resolution compared to panchromatic sensors.
- Offers a good balance between spatial and spectral resolution.
- Can capture visible, near-infrared, and sometimes shortwave infrared bands, enabling the identification of specific features like vegetation, water, and certain minerals.
- Applications:
- Widely used in agriculture for crop health monitoring and yield prediction.
- Land cover classification and vegetation mapping.
- Environmental monitoring and water resource management.
- Disaster assessment and damage mapping.
3. Hyperspectral Sensors:
- Description: Capture image data in a very large number of narrow spectral bands (often exceeding 100).
- Characteristics:
- Lower spatial resolution compared to panchromatic and multispectral sensors.
- Offers the most detailed spectral information for each pixel.
- Enables the identification and analysis of a vast array of materials and chemicals based on their unique spectral signatures.
- Applications:
- Mineral exploration and geological mapping.
- Precision agriculture and targeted irrigation management.
- Environmental monitoring and pollution detection.
- Scientific research in ecology, forestry, and atmospheric studies.
4. Ultraspectral Sensors:
- Description: Similar to hyperspectral sensors but with an even larger number of spectral bands and even higher spectral resolution.
- Characteristics:
- Extremely detailed spectral information across a wide range of wavelengths.
- Limited spatial resolution due to the complexity of capturing and processing such a vast amount of data.
- Applications:
- Advanced research applications in atmospheric science and climate studies.
- Detailed land surface analysis and vegetation characterization.
- Specialized environmental monitoring and hyperspectral imaging for specific scientific inquiries.
Remote Sensing Sensors

Remote sensing sensors can be broadly categorized into two types: passive and active. Passive sensors detect natural energy that is reflected or emitted from the Earth’s surface, typically from the sun. In contrast, active sensors use their own energy source to illuminate the Earth and measure the reflection. Examples of passive sensors include photogrammetric cameras and radiometers, while active sensors include RADAR and LiDAR systems. Each type of sensor has specific applications, depending on the nature of the data required and the environmental conditions under which it operates.
These rely on naturally occurring energy emitted or reflected from Earth, like sunlight or thermal radiation. Imagine them “seeing” what Earth radiates naturally.
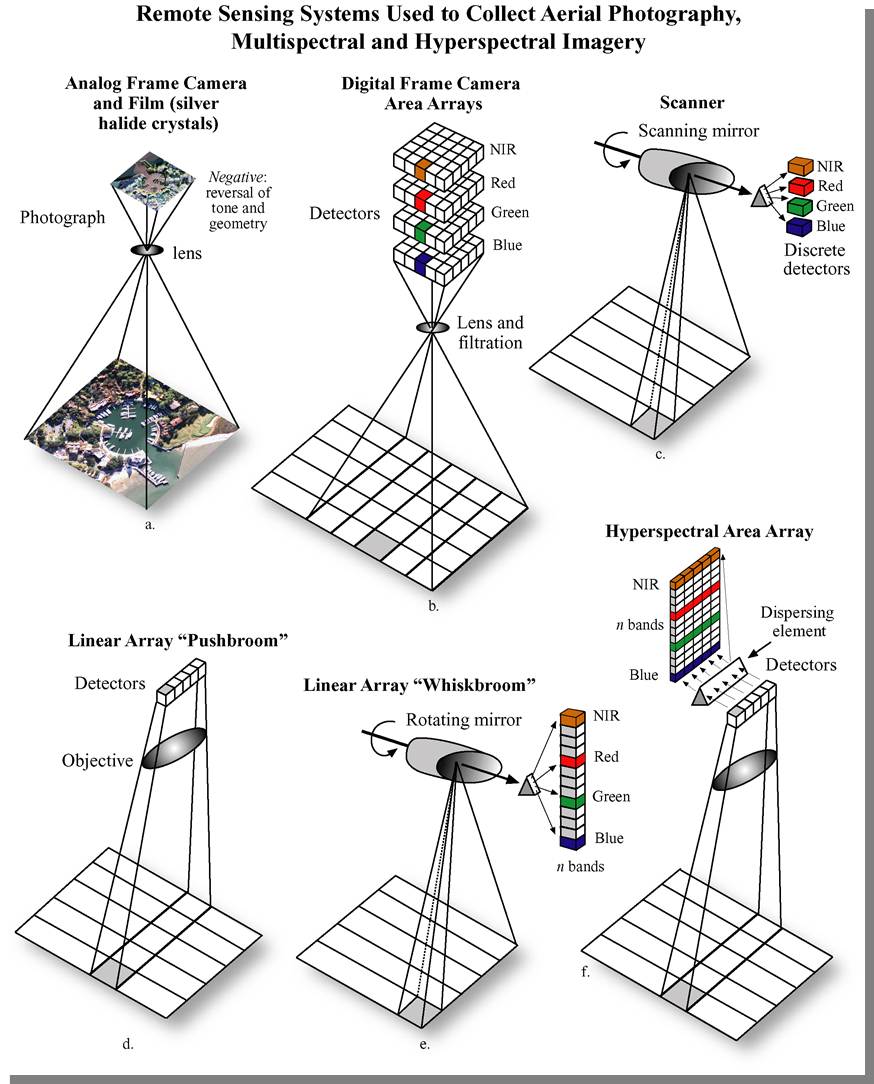
Multispectral Sensors: These capture images in multiple bands of the electromagnetic spectrum, like visible light and infrared, allowing us to differentiate between features like vegetation, water, and soil.
Hyperspectral Sensors: These divide the spectrum into much narrower bands, providing more detailed information about the chemical composition of materials, like identifying specific minerals or analyzing crop health.
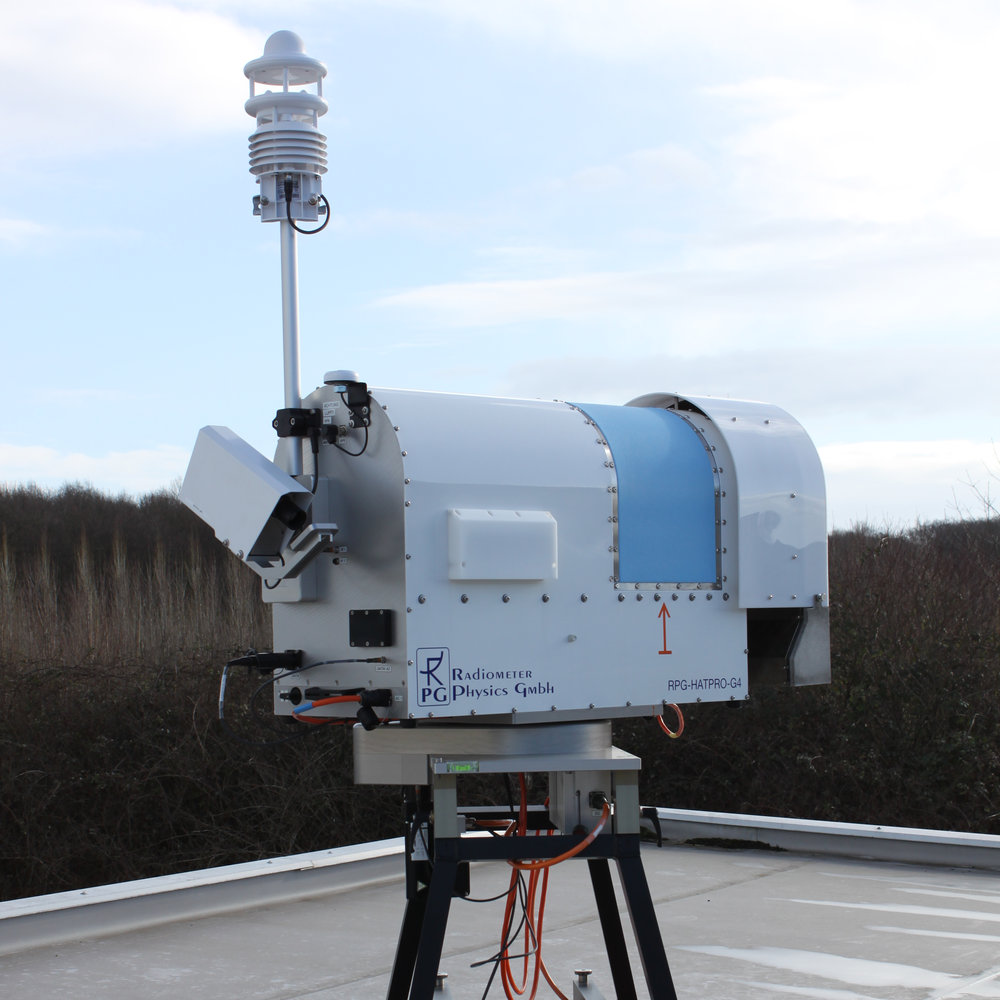
Radiometers: These measure the intensity of electromagnetic radiation, used to study temperature, soil moisture, and ocean salinity. They essentially “feel” the heat or radiation emitted by Earth.
These emit their own energy, like radar or lasers, and measure the reflected or scattered signal to create images or collect data. Imagine them “shining a light” on Earth and analyzing the bounce back.
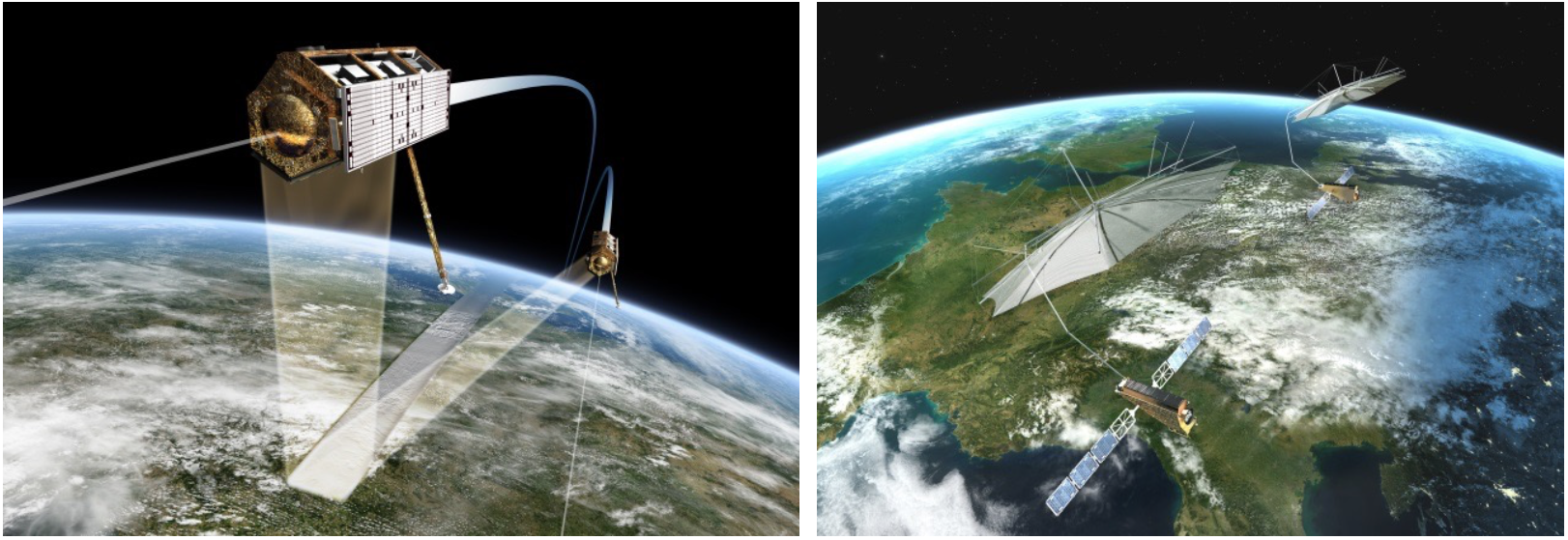
Radar: Sends out microwave pulses and records the reflected energy to map topography, track sea ice, and study vegetation structure. It’s like using radio waves to “see” through clouds or darkness
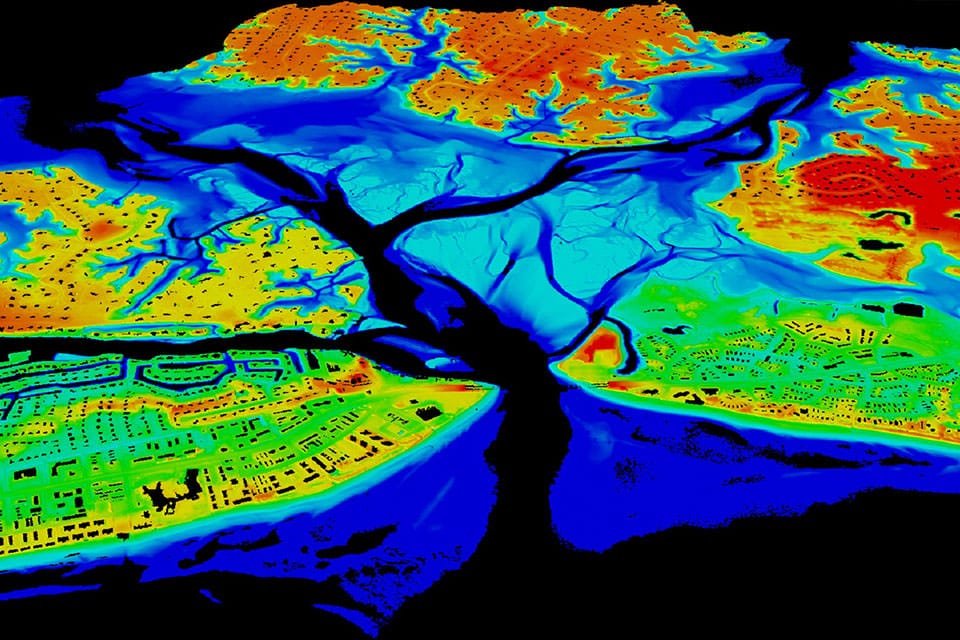
LiDAR (Light Detection and Ranging): Emits laser pulses and measures the time it takes for them to bounce back, creating highly accurate 3D maps of the Earth’s surface. Think of it as using lasers to precisely measure distances and build a 3D model of Earth.

SAR (Synthetic Aperture Radar): Creates high-resolution radar images by mimicking a large antenna using processing techniques. It’s like using advanced radar technology to get incredibly detailed pictures.
Satellite sensors are divided into two main categories: optical imaging sensors, which capture imagery in the visible and near-infrared spectrum, and radar imaging sensors, which utilize radar technology to penetrate clouds and darkness for versatile applications. Additionally, other sensors track satellite positions and provide vital atmospheric data.

Optical Imaging Sensors:
- Multispectral scanner: This sensor is designed to capture images by splitting the electromagnetic spectrum into discrete bands within the visible and near-infrared range. It operates by sequentially scanning these bands. Multispectral scanners are commonly employed for applications such as mapping land cover, assessing the health of vegetation, and monitoring water quality. They enable the identification of different land surface materials and the quantification of various environmental factors.
- Multispectral imagers: Similar to multispectral scanners, multispectral imagers are used to capture imagery across multiple spectral bands. However, they have the unique capability of simultaneously collecting data from all spectral bands, offering improved image quality and faster data acquisition. This simultaneous data capture is particularly beneficial for applications that require near-real-time responses, including disaster monitoring, emergency response, and rapid environmental assessments.
- VNIR (Visible and Near-Infrared): The VNIR sensor focuses specifically on the visible and near-infrared portions of the electromagnetic spectrum. It provides high-resolution imagery, offering detailed visual information about land cover, vegetation, and infrastructure. VNIR sensors are widely used in tasks that require visual interpretation and analysis, such as urban planning, agriculture, and forestry management.
- VIIRS (Visible Infrared Imaging Radiometer Suite): VIIRS is a multispectral sensor located aboard the Suomi National Polar-orbiting Partnership (Suomi NPP) satellite. It is instrumental in weather forecasting, climate change monitoring, and sea surface temperature measurements. VIIRS captures high-resolution data across multiple spectral bands, aiding meteorologists and climate scientists in understanding weather patterns and climate trends.
- OLCI (Ocean Land Colour Instrument): OLCI is an advanced multispectral sensor found on the Sentinel-3 satellite series. It specializes in measuring the colour of ocean and land surfaces, chlorophyll concentration in water bodies, and the presence of suspended sediments. OLCI contributes crucial data for studying ocean dynamics, water quality, and environmental changes, particularly in coastal areas.
- HyperCube: HyperCube is a hyperspectral sensor equipped on the WorldView-3 satellite. It stands out by capturing data in hundreds of narrow spectral bands. This high spectral resolution enables advanced analysis of materials, vegetation, and minerals. HyperCube’s detailed spectral data aids in identifying specific materials on Earth’s surface, making it valuable for mineral exploration, environmental monitoring, and precision agriculture.
- SHySIS (Spectro-Hypercube System): As part of the EnMAP (Environmental Mapping and Analysis Program) mission, SHySIS is a hyperspectral sensor designed to provide unprecedented spectral detail. It offers highly specific spectral information for geological mapping, environmental monitoring, and agricultural assessments. SHySIS’s rich spectral data allows scientists to study the composition and health of ecosystems in great detail.
Radar Imaging Sensors:
- SAR imager (Synthetic Aperture Radar): SAR operates by emitting its microwave pulses and recording the resulting echoes. It can see through clouds and darkness, making it highly valuable for disaster monitoring, ice mapping, maritime surveillance, and terrain analysis. SAR provides detailed images of surface features by measuring the time it takes for radar signals to bounce back from the Earth’s surface.
- X-band SAR imager: X-band SAR imagers are specialized in using X-band frequency microwaves to capture high-resolution images with remarkable sensitivity to surface features. This makes them ideal for tasks such as flood mapping, infrastructure damage assessment, and monitoring land subsidence. However, X-band SAR can have limitations in areas with dense vegetation cover or rugged terrain due to scattering effects.
- ASCAT (Advanced Scatterometer): ASCAT measures ocean wind speed and direction over the ocean surface using radar scatterometry. Its data is essential for weather forecasting and oceanographic studies, contributing to our understanding of atmospheric and oceanic conditions. ASCAT’s radar signals interact with the ocean surface to provide valuable information on wind patterns and sea surface conditions.
- SRAL (Sentinel-3 Radar Altimeter): SRAL is a radar altimeter system onboard the Sentinel-3 satellite series. It specializes in measuring sea surface height with exceptional precision. The data generated by SRAL is crucial for models of ocean circulation, climate change studies, and the monitoring of sea level rise. SRAL provides accurate information about the elevation of the ocean surface and is vital for understanding Earth’s changing climate.
Other Sensors:
- DORIS (Doppler Orbitography and Radiopositioning Integrated System): DORIS is responsible for tracking the precise position of satellites in orbit. Its data is essential for ensuring accurate Earth surface measurements and the proper functioning of various satellite missions. DORIS achieves this by using Doppler shifts in radio signals transmitted between ground stations and satellites to calculate satellite positions with exceptional accuracy.
- CrIS (Cross-track Infrared Sounder): CrIS is an advanced sensor that measures atmospheric temperature and composition profiles for weather forecasting and climate monitoring. It operates by collecting infrared radiation emitted by Earth’s atmosphere, providing vital information about temperature and the distribution of various atmospheric gases.
- ATMS (Advanced Microwave Scanning Radiometer): ATMS is a microwave radiometer that complements CrIS by providing additional atmospheric temperature and moisture data. This enhances the accuracy of weather forecasting models and contributes to our understanding of atmospheric processes
Summary of Active and Passive Remote Sensing Sensors and their Applications
Sensor Type | Description | Applications | Active/Passive |
Multispectral Scanner | Captures images in multiple predefined spectral bands | Land cover classification, vegetation analysis, environmental monitoring, mineral exploration, precision agriculture | Passive |
SAR Imager | Uses microwave pulses to create high-resolution images | Urban monitoring, infrastructure assessment, disaster management, flood mapping, deforestation detection, ice and snow monitoring | Active |
X-band SAR Imager | High-resolution X-band SAR for detailed surface features | Urban infrastructure monitoring, forest structure analysis, crop health assessment, flood inundation mapping | Active |
VIIRS | Provides visible and infrared imagery for weather forecasting | Cloud analysis, sea surface temperature measurement, land surface temperature mapping, vegetation monitoring | Passive |
CrIS, ATMS, OMPS | Measure atmospheric temperature, composition, and ozone concentration | Weather forecasting, climate change monitoring, air quality analysis, volcanic eruption detection | Passive |
MVIRI, IASI, GRAS | Gather meteorological data for weather forecasting and atmospheric studies | Cloud characterization, atmospheric temperature and humidity profiles, wind speed and direction measurement | Passive |
ASCAT | Measures wind speed and direction over the ocean surface | Ocean colour analysis, chlorophyll concentration mapping, phytoplankton bloom detection, sea surface temperature measurement | Active |
DORIS | Tracks satellite position using ground-based beacons | Precise satellite orbit determination, global navigation satellite system (GNSS) support, geodetic studies | Passive |
Poseidon-4, LaRC, ALTIKA | Measure sea surface height, waves, and currents | Ocean weather forecasting, wave modelling, climate research, air-sea interaction studies | Active |
OLCI, SLSTR | Provide multispectral imagery, sea surface temperature, and height data | Ocean color analysis, chlorophyll concentration mapping, phytoplankton bloom detection, sea surface temperature measurement | Passive |
SRAL | Measures sea surface height using radar | Ocean topography mapping, ice sheet volume calculation, coastal erosion monitoring, tide prediction | Active |
GF1, SWIHR | Gather oceanographic data on sea surface temperature and salinity | Ocean salinity mapping, sea ice monitoring, water quality assessment, fishery management | Passive |
MWRI | Measures microwave radiation from the Earth’s surface or atmosphere | Sea surface wind speed and direction measurement, soil moisture estimation, snow cover mapping, ice thickness monitoring | Active |
HyperCube, VNIR, WV-3 | Hyperspectral and high-resolution imaging for mineral exploration, vegetation analysis, and urban planning | Geological mapping, mineral identification, vegetation stress detection, precision agriculture, urban infrastructure inspection | Passive |
VNIR, SIF | Analyze vegetation stress, mineral deposits, and land use changes | Crop health monitoring, forest fire detection, mineral exploration, vegetation phenology studies, land degradation assessment | Passive |
SHySIS, OKSI, APEX | Provide high-resolution and multispectral data for geological mapping, agriculture, and environmental monitoring | Geological resource exploration, soil characterization, vegetation mapping, precision agriculture, environmental impact assessment | Passive |
Sensor Resolutions
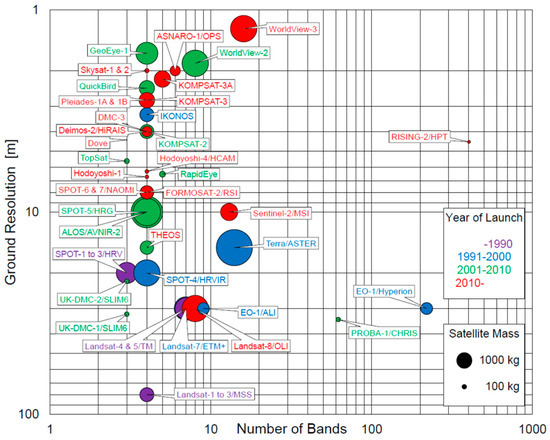
Sensor resolution in remote sensing is the measure of a sensor’s ability to capture detail in the data it collects. It determines how finely a sensor can represent or measure something, be it an object, area, or phenomenon, from a distance. High-resolution sensors capture finer details, while lower-resolution sensors provide a more general view. This resolution affects the quality and effectiveness of the data for various applications.
Four types of resolutions are…
Concept: As mentioned previously, spatial resolution defines the smallest feature on the ground a sensor can distinguish. Imagine peering through a microscope; the higher the magnification, the finer the detail you see. Similarly, lower spatial resolution values (e.g., 10m) represent higher resolution, allowing you to see individual trees or buildings. Conversely, higher values (e.g., 1km) offer broader overviews at the expense of details.
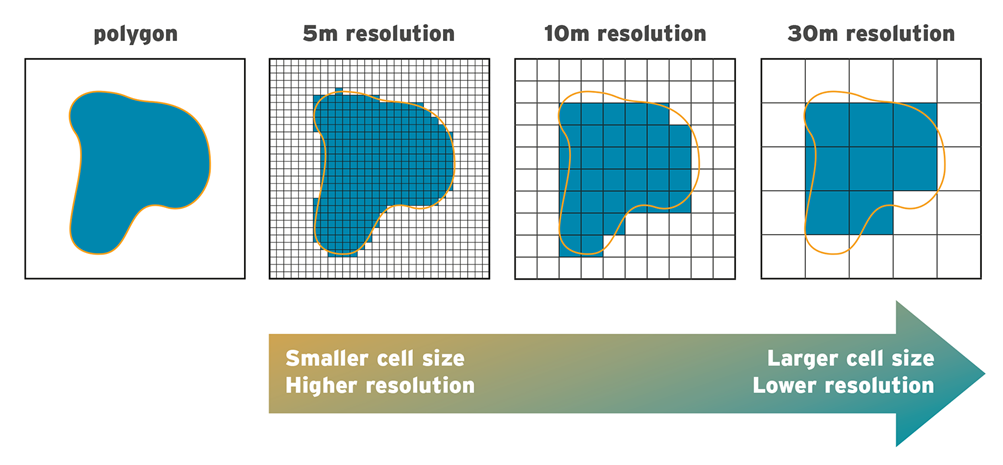
Mechanics: Several factors influence spatial resolution, including:
- Sensor aperture: Smaller apertures focus light onto a smaller area, enabling finer detail capture.
- Detector array density: More densely packed detectors allow for smaller individual pixels, representing smaller ground areas.
- Field of view (FOV): A narrower FOV concentrates the sensor’s focus on a smaller area, increasing spatial resolution for that area.
Additional Details:
- Types of Spatial Resolution: Panchromatic images record brightness in a single band, while multispectral and hyperspectral images offer varying levels of detail by capturing data in multiple bands.
- Ground Sampling Distance (GSD): GSD represents the actual size of each pixel on the ground, directly related to spatial resolution. A 10m GSD image means each pixel represents a 10m x 10m area on the ground.
- Trade-offs: Higher spatial resolution often comes at the cost of a smaller coverage area, increased sensor cost, and potentially lower temporal resolution due to data processing limitations.
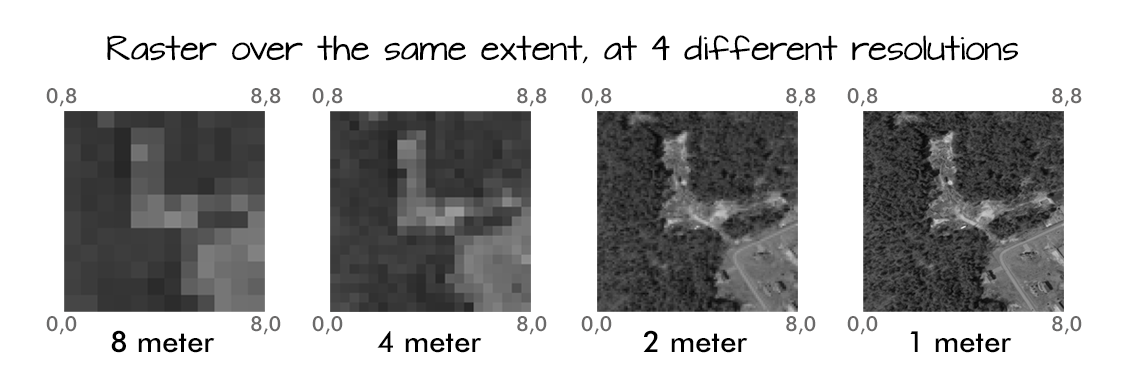
Concept: Spectral resolution defines the range of wavelengths or colours a sensor can detect. Think of it like the number of “crayons” in a box; more crayons allow for more intricate detail and differentiation. Sensors capture varying ranges of the electromagnetic spectrum, from visible light (red, green, blue) to invisible wavelengths like infrared and microwaves. Different materials have unique spectral signatures, reflecting or emitting radiation at specific wavelengths.
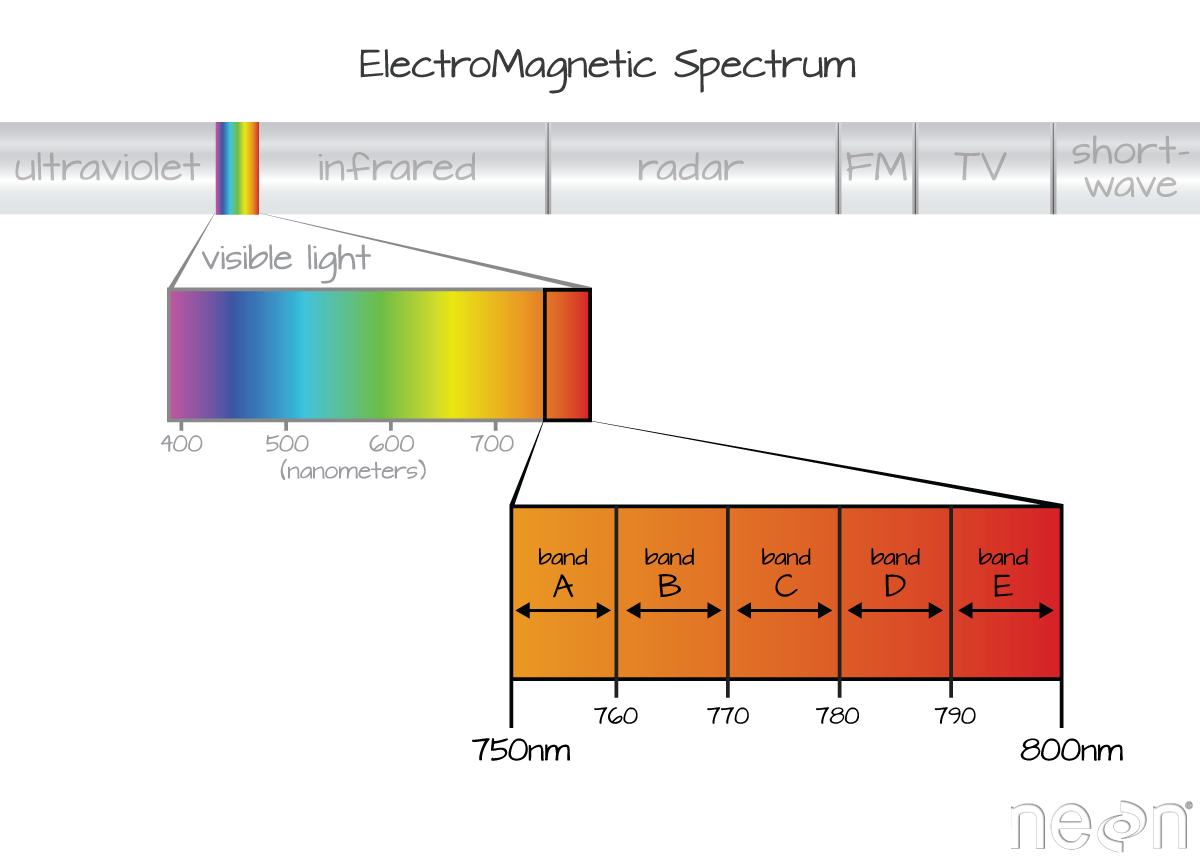
Mechanics: Sensors employ filters or detectors sensitive to specific wavelength ranges. Multispectral sensors typically capture data in several discrete bands, while hyperspectral sensors record continuous spectra with hundreds of bands, offering a much richer “palette” of colours and information.
Additional Details:
- Band Selection: The choice of specific bands influences the types of information a sensor can capture. For example, near-infrared bands highlight vegetation health, while thermal infrared bands detect heat signatures.
- Spectral Indices: Scientists often combine data from multiple bands to create spectral indices, calculated values that enhance specific features or properties of interest.
- Trade-offs: Higher spectral resolution often comes at the cost of lower spatial resolution due to data volume constraints, increased sensor cost and complexity, and more demanding data processing needs.
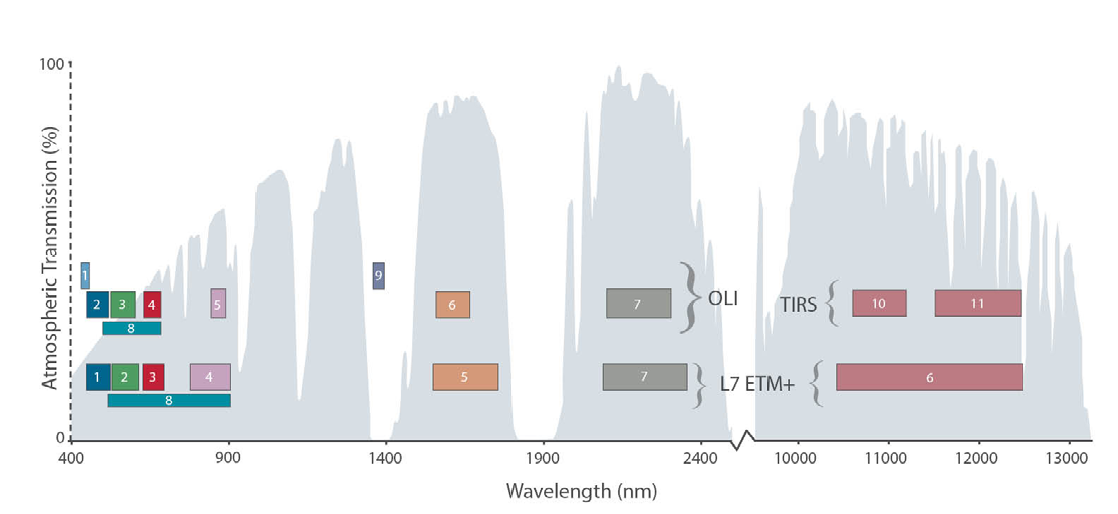
Here’s a table for Sentinel-2, a multispectral imaging satellite:
Band Number | Description | Wavelength (µm) | Spectral Resolution (nm) | Spatial Resolution (m) |
1 | Coastal aerosol | 0.443 – 0.490 | 47 | 60 |
2 | Blue | 0.490 – 0.560 | 70 | 10 |
3 | Green | 0.560 – 0.665 | 105 | 10 |
4 | Red | 0.665 – 0.705 | 40 | 10 |
5 | Vegetation red edge | 0.705 – 0.740 | 35 | 20 |
6 | Vegetation red edge | 0.740 – 0.783 | 42 | 20 |
7 | Vegetation red edge | 0.783 – 0.865 | 82 | 20 |
8 | NIR | 0.842 – 0.877 | 35 | 10 |
8A | Narrow NIR | 0.865 – 0.885 | 20 | 20 |
9 | Water vapor | 0.945 – 1.020 | 75 | 60 |
10 | SWIR – Cirrus | 1.360 – 1.390 | 30 | 60 |
11 | SWIR | 1.565 – 1.655 | 90 | 20 |
12 | SWIR | 2.100 – 2.280 | 180 | 20 |
Concept: Temporal resolution refers to the frequency with which a sensor revisits the same area. Imagine having a set of snapshots taken at different intervals; the more frequent the snapshots, the better you can track changes over time. Measured in days, weeks, or even hours, temporal resolution determines the time span between subsequent observations.

Mechanics: Satellites follow specific orbital paths, revisiting certain areas at designated intervals. The sensor’s coverage pattern and data transmission capabilities also influence temporal resolution.
Additional Details:
- Revisit Time: Specific satellites offer varying revisit times depending on their orbits and sensor capabilities. Some, like Sentinel-2, revisit areas every 5 days, while others, like Landsat, have a 16-day revisit cycle.
- Applications: High temporal resolution is crucial for monitoring rapid changes like floods or volcanic eruptions, while lower resolutions are sufficient for studying slower trends like deforestation or seasonal vegetation changes.
- Trade-offs: Higher temporal resolution often comes at the cost of lower spatial resolution due to limitations in sensor design and data acquisition capabilities. Additionally, frequent data acquisition and processing can be resource-intensive and costly.
Temporal resolution of some popular satellites
Satellite | Sensor | Revisit Time | Applications |
Landsat-8 | OLI & TIRS | 16 days | Land cover change, vegetation monitoring, agriculture, forestry |
Sentinel-2 | MSI | 5 days (2 satellites combined) | Vegetation health, water quality, urban planning, disaster management |
WorldView-3 | PAN & MS | 1-3 days (off-nadir) | High-resolution mapping, infrastructure monitoring, resource exploration |
PlanetScope | Falcon | Daily | Global change monitoring, near real-time observations, environmental monitoring |
RapidEye | ReRapid | 5 days | Rapid response to disasters, deforestation detection, land-use planning |
MODIS | Aqua & Terra | 1-2 days | Global climate change, weather forecasting, ocean monitoring |
NOAA-GOES 16 & 17 | ABI | 10-15 minutes | Severe weather monitoring, cloud tracking, volcanic eruptions |
Sentinel-1 | C-SAR | 6 days (SAR) | Maritime traffic monitoring, ice mapping, flood monitoring |
SkySat | SAR | Daily (multiple satellites) | Maritime security, disaster response, infrastructure monitoring |
Concept: Radiometric resolution defines the level of detail in the brightness (intensity) of each pixel in an image. Think of it like the number of shades you can use to depict light and shadow. Higher radiometric resolution allows for finer gradations and subtle differences in intensity, revealing nuances that might be masked in lower resolutions. Measured in bits (e.g., 8-bit, 12-bit, 16-bit), higher numbers indicate greater sensitivity and finer brightness variations.

Mechanics: Factors influencing radiometric resolution include:
- Sensor sensitivity: More sensitive detectors can capture a wider range of light intensity values.
- Signal processing: Advanced algorithms can enhance the signal-to-noise ratio, improving the accuracy and detail of brightness information.
- Calibration: Accurate



Different kinds of sensor resolution (Source: Mahmood et al., 2023)
Major Remote Sensing Platforms
This table provides a comprehensive overview of various Earth observation satellites across diverse organizations and applications.
Resolution: Spatial, Spectral, Temporal, Radiometric Resolution. | Satellite Series | Launch Date | Orbit Type | Orbit Altitude | Number of Satellites | Sensors | Spectral Resolution | Spatial Resolution | Temporal Resolution | Radiometric Resolution | Key Applications |
Disaster Monitoring | |||||||||||
Israel | DMOSAT-1 | 2009 | Sun-synchronous | 700 km | 1 | Multispectral scanner, SAR imager | Visible, near-infrared, radar | 2.5m, 10m (radar) | 5 days | NA | Disaster response, emergency management, infrastructure monitoring |
Italy | COSMO-SkyMed | 2007 (first launch) | Sun-synchronous | 450 km | 4 (operational) | X-band SAR imager | N/A | 3m – 10m | 12 days | NA | Flood and earthquake monitoring, landslide detection, maritime surveillance |
Germany | RapidEye | 2008 (first launch) | Sun-synchronous | 630 km | 5 (1 operational) | Multispectral imagers | Visible, near-infrared, red-edge | 5m | 4 hours (combined constellation) | 10-bit | Near-real-time disaster monitoring, deforestation detection, infrastructure damage assessment |
Climate and Atmospheric Studies | |||||||||||
NASA/NOAA | Suomi NPP | 2011 | Sun-synchronous | 824 km | 1 | VIIRS, CrIS, ATMS, OMPS | Visible, near-infrared, infrared, ultraviolet | 375m, 1.4km (infrared) | 1 day | VIIRS: 10-bit for VNIR and MVIRI, 12-bit for I-bands CrIS: 14-bit for all bands ATMS: 12-bit for all bands OMPS: 10-bit for all bands | Weather forecasting, climate change monitoring, atmospheric composition analysis |
EUMETSAT | MetOp-A and B | 2006, 2012 | Geostationary | 35,786 km | 2 | MVIRI, IASI, GRAS, ASCAT | Visible, near-infrared, infrared, radar | 1.25km, 4km (infrared) | Continuous observation (geostationary) | MVIRI: 10-bit for all bands IASI: 14-bit for all bands GRAS: 12-bit for all bands ASCAT: N/A (not applicable, wind scatterometer) | Weather forecasting, storm tracking, air quality monitoring |
Oceanographic Applications | |||||||||||
NASA/CNES/EUMETSAT | Jason-3 | 2016 | Near-polar | 1,336 km | 1 | DORIS, Poseidon-4, LaRC, ALTIKA | Radar altimetry | N/A | 10 days | NA | Global sea level measurement, ocean circulation monitoring, climate change studies |
ESA | Sentinel-3 | 2016 | Sun-synchronous | 850 km | 2 | OLCI, SLSTR, SRAL, DORIS | Visible, near-infrared, infrared, radar | 300m, 1km (infrared) | 27 days | OLCI: 12-bit for all bands SLSTR: 12-bit for VNIR and SWIR, 14-bit for TIR SRAL: N/A (radar altimeter) | Ocean topography, sea surface temperature, sea surface salinity, marine pollution monitoring |
CNSA | HY-2A and B | 2011, 2018 | Sun-synchronous | 830 km | 2 | GF1, MWRI, SWIHR, SCAT | Visible, near-infrared, microwave | 250m, 500m (microwave) | 4 days | GF1: 10-bit for VNIR and SWIR, 12-bit for MWIR MWRI: 12-bit for all bands SWIHR: 10-bit for all bands SCAT: N/A (not applicable, wind scatterometer) | Ocean wind speed, wave height, sea surface salinity, ice monitoring |
Hyperspectral Imaging | |||||||||||
Maxar Technologies | WorldView-3 | 2014 | Sun-synchronous | 496 km | 1 | HyperCube, VNIR, WV-3 | Hyperspectral, visible, near-infrared | 3.7m (hyperspectral), 0.34m (VNIR) | 1 day | HyperCube: 14-bit VNIR: 12-bit WV-3: 12-bit | Mineral exploration, environmental monitoring, precision agriculture, vegetation mapping |
CNES | HySIS | 2018 | Sun-synchronous | 694 km | 1 | VNIR, SIF | Visible, near-infrared, shortwave infrared | 1.5m, 75m (SIF) | 26 days | VNIR: 12-bit SIF: 16-bit | Environmental studies, vegetation mapping, mineral exploration, pollution detection |
Germany | EnMAP | 2024 (planned) | Sun-synchronous | 678 km | 1 | SHySIS, OKSI, APEX | Hyperspectral, visible, near-infrared | 6m (hyperspectral), 20m (VNIR) | 26 days | SHySIS: 14-bit OKSI: 12-bit APEX: 16-bit | Geological mapping, |